How Technology is Preventing Market Abuse in Fintech
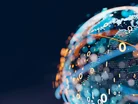
When it comes to detecting and stopping misconduct or non-compliant behavior, surveillance teams have to act as detectives – organizing large volumes of amounts of data, discovering the truth within it and acting on what they find. Teams have the difficult task of essentially finding the needle in the haystack, just to ensure the few instances of market abuse are stopped and reported.
Yet, despite the fact that proactive monitoring of emails has been a regulatory requirement for quite some time, many organizations rely on antiquated methods of surveillance. These approaches depend on the use of certain search terms or lexicons to identify potential issues and alert compliance teams. This legacy approach to detecting misconduct causes a flood of mostly not-relevant alerts — sometimes hundreds of thousands a day — that must be dealt with by reviewers.
The problem with words
Just flagging risky words is not a fool-proof system for detecting misconduct. As an example, in everyday conversation, I may tell someone I guarantee they will have a good time at the football game or the party this weekend. But if I’m a financial advisor communicating with a client, I can’t say that I guarantee something — especially a certain stock going up tomorrow because it’s a risky asset and there's a real chance it goes down.
So, when a legacy surveillance system monitoring emails sees the word “guarantee'' in a message or other communication, it flags the message for review without understanding the context. The problem is that we use common words, like guarantee, in other contexts every day that have nothing to do with compliance risks or financial markets. When relying on just lexicons, you’re mostly just reviewing junk communications that have nothing to do with the risks for which you are looking.
An alert factory
The main goal, when we’re talking about communication surveillance in the financial industry, is to prevent issues before they become real problems. We hear a lot about misconduct — which can refer to things like money laundering, collusion or insider trading — in financial services, and many of these could have been quickly detected with some proactive monitoring. But in order to do this, surveillance platforms need to drastically reduce false positives so they have the bandwith to actually investigate meaningful risks.
Historically, surveillance has been more of an alert factory. Organizations have an army of reviewers who spend their days looking over a mountain of alerts — ones that are mostly irrelevant. They review, escalate and repeat day after day, mostly sifting through junk. When you have so many false positives, an army of people wastes a lot of time reviewing and clearing those alerts.
A better approach
It starts with two areas of technology that are booming today — artificial intelligence (AI) and machine learning. The goal is to make all communications, including email, chat and audio searchable leveraging the power of AI and machine learning to surface only the most relevant data to reviewers.
Modern communication surveillance platforms seamlessly pull communications from every enterprise communication system system to ensure you have full coverage. An intuitive user interface with advanced workflow configurability, adapts to your organizational workflows securely in the cloud. Machine learning is always running in the background and gets smarter with every review to only serve up the riskiest content and reduce false positive alert volumes.
When an alert comes through that is valid and calls for a true investigation, the reviewers can use the same modern system to launch that investigation with integrated case management.
Email thread deduplication is another key feature — which locates old email content within new emails, checks that it was already analyzed and prevents it from generating another alert. The data cleansing capabilities of modern systems identify email headers, signatures and confidentiality disclaimers. They prevent alerts from being generated on this duplicative or non-authored content, so reviewers are only notified of the relevant portion that was written by the sender. Reviewers also can see the content that was cleared, which provides complete transparency to how the machine learning is working.
With all the great advancements in surveillance and the modern systems available, the obvious question is why haven’t all organizations moved to this future state? One answer may be the historic lack of transparency of these models.
Lexicons, while ineffective, are easily explained (e.g., this email was alerted on because it had this word in it). There is a great deal of fear in the industry about transitioning to machine-learning models because you now have to defend it. As we move forward, it’s the surveillance innovators’ responsibility to make sure that all AI is transparent and explainable, and ultimately defensible to regulators. Tech companies will continue to invest in these new technologies to help make processes more exact and efficient and help surveillance teams catch misconduct. Meanwhile, users will be able to discover the truth in the data and act on it to help combat manipulation.
About the author: Jordan Domash is Relativity's general manager of Relativity Trace. He guides a focused team in the development of this tool, built on the Relativity platform, for proactive compliance monitoring, supporting engineering, marketing, and sales.